32 is the Magic Number: Deep Learning's Perfect Batch Size Revealed!
- Jainam Shroff
- Mar 6
- 1 min read
Batch size has a massive impact on model training and performance!

While large batch sizes can improve computational parallelism, they may degrade model performance. In contrast, small batch sizes enhance the model's generalization ability and require less memory. Given these advantages—better generalization and reduced memory footprint—using smaller batch sizes for training makes sense.
Yann LeCun suggests in his tweet that a batch size 32 is optimal for model training and performance.
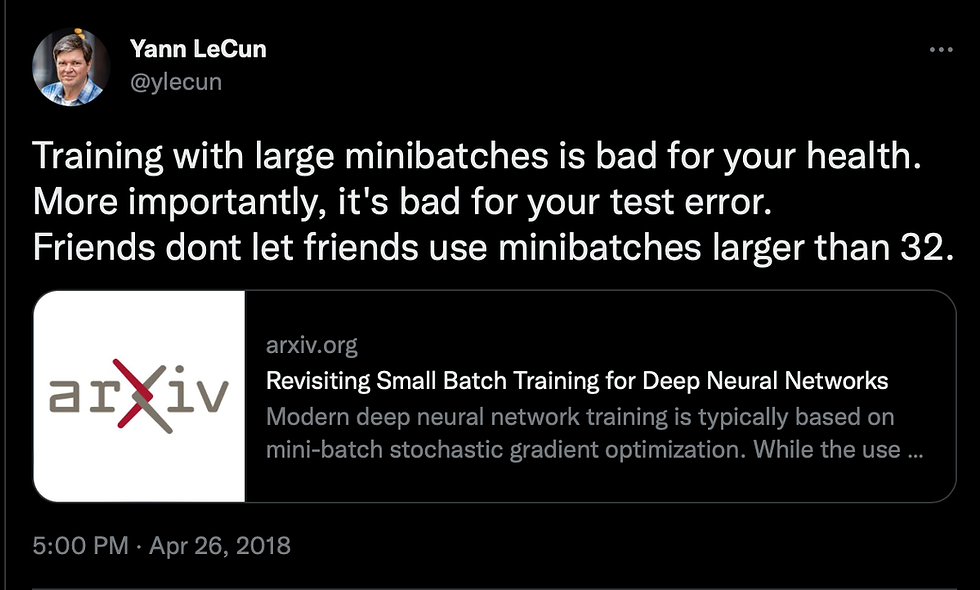
In the paper Revisiting Small Batch Training for Deep Neural Networks, researchers conducted experiments across multiple datasets—CIFAR-10, CIFAR-100, and ImageNet—to determine the optimal batch size. Their tests examined how different batch sizes affect training time, memory usage, and performance. The study found that batch sizes between 2 and 32 outperform larger sizes in the thousands.
Why does a smaller batch size of 32 perform better than a larger one?
A smaller batch size enables more frequent gradient updates, resulting in more stable and reliable training.
Conclusion: Friends, don't let friends use batch sizes larger than 32.
Comentarios